Generative artificial intelligence, in the form of LLM-powered "superbots,"
Depending on what you read, AI — usually referring to the
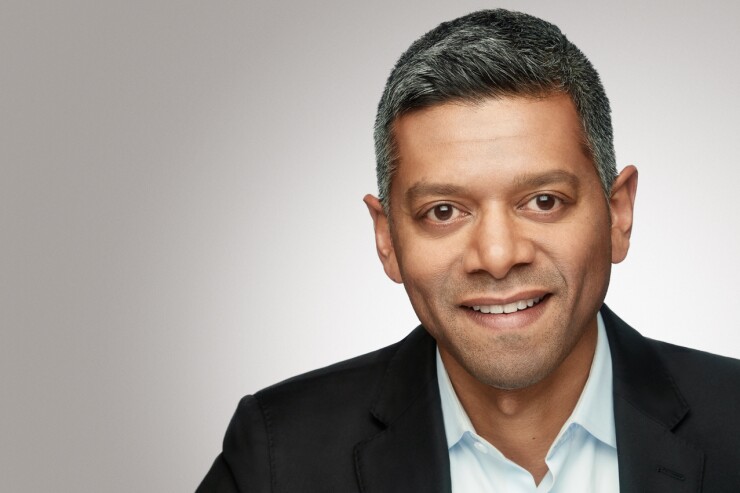
As the founder of a wealth technology fintech firm who works with and advocates for AI every day, I, of course, have a different perspective.
My company is in the business of solving business problems for wealth and advisory firms; AI is just the tool we use to make it happen. But since we emphasize the role of AI, we do a lot of explaining and myth-busting — not just about our technology, but around framing how advisors and technologists should be talking about AI in the wealth space.
Here are some of the questions I get asked and how I respond.
Isn't AI unproven? Doesn't it make a lot of mistakes? How can I trust its answers?
Questions of this nature usually come from people who have read the latest story about ChatGPT or LaMDA or some other large language model. First, it's important to remember that these forms of AI are designed to replicate neural networks in communicating ideas.
Next, the algorithms, data inputs and outputs used in LLMs are fundamentally different from those we use to answer questions in wealth management. How AI is used in wealth management varies and will continue to evolve as new applications are developed. Some companies will focus on learning and predicting how markets move; others may design programs to answer technical questions from investors. At TIFIN Wealth, we focus entirely on using AI to empower organic growth at advisory firms.
We do this with what I call "precision" AI — highly specialized applications that are fed only verified data, use custom-built algorithms, and are tasked with answering very specific questions such as "How likely are specific clients to aggregate more of their portfolio with me?" or "Which of my clients know people who would be good prospects, and who are those prospects?" If the data and feedback loop are both well designed, the algorithm learns as it is designed to. If the questions are specific and quantifiable, the kinds of humorous and/or scary mistakes one attributes to AI are not a concern.
Aren't these questions advisors and business development people can arrive at by themselves?
The short answer is yes, but I propose that AI can do it better. The wealth management space is particularly well served by AI simply because it is a data-rich environment, both in terms of the amount of data and the number of discrete data sources required to ask and answer these questions. This is why computer models have been a part of wealth management as long as there have been computers. We are comfortable with the idea of quants running complex models using market data, and that field has only gotten more advanced with AI. Since it learns, the model doesn't need to be constantly updated. For wealth management firms, we are proposing that allowing AI to digest and interpret data from different sources — company records, marketing engagement data, vendor data and social networks — is much more efficient and predictive than asking a human to do the same task.
The learning aspect is another reason AI is better than either humans or static decision-tree models that have been used in the past. With real-time data feeding the learning of the algorithm, it won't get out of date or draw outdated conclusions as the marketplace evolves. We've established our service with multiple clients and although the initialization looks the same, the results down the road are different because the model is learning from different data sources and answering different questions.
Shouldn't firms be building their own proprietary systems?
I think — no surprise here – an external precision AI partner will serve wealth management firms better.
First, there's the challenge of talent. Wealth firms have many, many smart technologists, but the people writing cutting-edge algorithms tend to be industry-agnostic and less likely to focus on a single business. In our firm around half of our people are focused just on product, engineering and data science. And, unlike our clients, we don't have to manage investment products or advisors. We specialize, solve problems and set clients up for success.
Second, external firms have larger amounts of data to use to train and refine the algorithm. While, of course, a firm's data is proprietary and never shared across clients, learnings from one source can be applied to improve the algorithm used by another. The more everyone succeeds, the more questions we answer correctly, the more the technology benefits everyone — a virtuous cycle.
What's next for wealth management and precision AI?
The most exciting thing about being at the intersection of AI and wealth management is that there is so much more to come. The appetite for and interest in AI has exploded and — misconceptions aside — we are finding ourselves asking questions about wealth management we never imagined could be answered.
Each new engagement teaches us something new and we continue to evolve ourselves. We're just as curious as you to see how precision AI will grow.