Just about every financial services company that I talk to wants to tap big data to unlock more accurate market predictions, minimize risk and personalize product offerings and advice. Yet despite significant investments in analytics, many of these companies still rely too much on intuition and gut instinct while their big data projects flounder. What’s going on? All too often, there’s a disconnect between what the business needs and what analytic implementations actually deliver.
Here’s a common issue that I see: business stakeholders don’t get involved early enough in analytics initiatives, assuming that IT experts will magically lead the way to big data nirvana. This is backwards, as every technical consideration is ultimately dependent on what the wealth managers, risk analysts, traders and other key front-line stakeholders in the organization need to achieve. When it comes to big data and financial services, here’s what the data side of the house wishes the business side would understand:
● Analytics is an enabler, not an end goal. All too often, analytics projects are initiated because of a vague notion that, “We need to do something to capitalize on all this data.” Analytics is not an objective. Rather, it is enabler that empowers business stakeholders to achieve their objectives in their specific areas of expertise, whether they are providing investment advice, analyzing credit risks, making buy/sell decisions, etcetera. Many companies get tripped up because they focus first on the “how” — analytical infrastructure and tools — before defining “why” — “Why are we doing this in the first place?” Just as you wouldn’t start constructing a building without first knowing its purpose (Shopping mall? Apartment complex? Hospital?), it makes no sense to “just get started” building databases and algorithmic models before clearly outlining a tangible business objective.
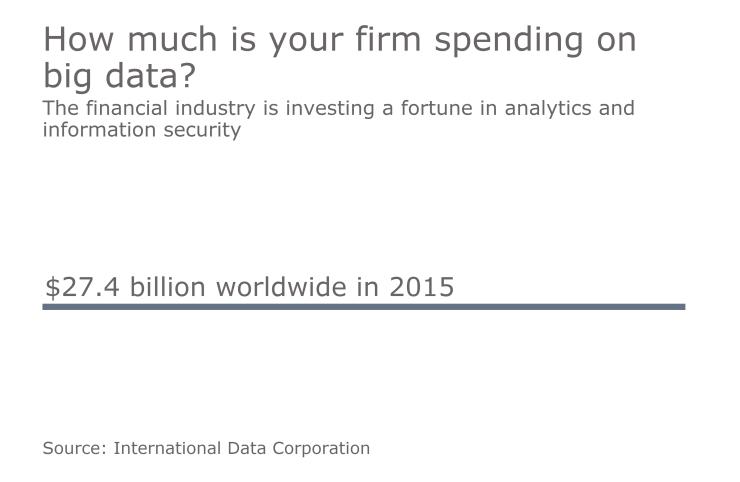
● Data scientists are not magicians. Like any practice within an organization, big data analytics involves a lot of trial and error, the constant honing of processes for continuous improvement and hard work. While it would be great if, armed with the right algorithm, data scientists could capture game-changing intelligence as easily as waving a magic wand, this is hardly ever how it works in the real world. End users would do well to educate themselves about how data can most effectively be leveraged within the context of their specific business practices, so that they can set realistic, achievable objectives for the data experts. At the end of the day, analytics does not replace human expertise. It simply helps arm domain experts with more information that they can use to make the best possible decisions.
● You can’t spin garbage into gold. The best analytics implementation in the world is not going to solve the problem of bad data. Financial services may be awash in torrents data — in the form of market transactions, customer profiles, risk and performance analyses, trading data and more — but within most organizations, information sources are often siloed across a dizzying array of departments and business practices. Harnessing it all successfully is messy and complex. For big data analytics to be successful, the raw material needs to be accurate and easy to access. Common data quality issues such as siloed information, inconsistent taxonomies and inadequate cleansing and preprocessing must be addressed first, before moving on to analytics.
● Insight must drive action. When it comes to analytics, “insight” is one of those buzzwords that’s tossed around a lot. But to be truly valuable, insight captured using data analytics must drive tangible actions — whether that means prompting a wealth manager about which clients to call on a given day based on the opportunities uncovered through the data, or alerting trading desks and risk groups on possible impending credit events or market shocks. Getting to the “action” end-point requires analytics that go beyond surface insights and visualizations and that are embedded into the tools and technologies that business stakeholders regularly use. Data experts need to know what these end-points are, so that analytics can be pushed to business users, whether through a CRM system, risk analysis application, or other critical end-point.
● Moving data is tough. As we all know, the world of financial services involves massive data flows streaming in from multiple venues and sources. NYSE, CME, or BATS produce terabytes of tick data a day. Moving data around so it can be analyzed is just as challenging as moving mountains. This is the number one issue that kills big data projects in their tracks, because of the data quality, storage and integration issues involved. A better strategy is to bring the analytics to the data, not the other way around. This is again why it is so important that the business be able to clearly define their objectives. When the data side of the house knows what the business needs, it can focus on layering analytics over the most relevant existing sources of data, rather than spend months figuring out how move billions of rows of data from one system to another, like Sisyphus pushing a boulder uphill.
Leveraging data and analytics within financial services is an ongoing practice that, at the end of the day, needs to be woven into the everyday activities of the business. The reality is that there is no finish line, no point in time at which one could deem an analytics project complete. As new data sources become available and business priorities evolve, analytics will need to answer different questions and address changing objectives. To be successful on an ongoing basis, the data side of the house needs constant feedback and guidance from the business. What’s working? What isn’t? How are needs evolving? Flexibility, agility and practicality will ultimately serve the business better than myopically focusing only on technical considerations in the search for an (ultimately unrealistic) “perfect” solution.